class: center, middle # Visualización de datos:<br>*ggplot2 strikes back* ### Análisis estadístico utilizando R <img src="https://raw.githubusercontent.com/rstudio/hex-stickers/master/PNG/tidyverse.png" width="10%" /><img src="https://raw.githubusercontent.com/rstudio/hex-stickers/master/PNG/ggplot2.png" width="10%" /> UNQ UNTreF CONICET Ignacio Spiousas [<svg viewBox="0 0 496 512" style="height:1em;position:relative;display:inline-block;top:.1em;fill:#A42339;" xmlns="http://www.w3.org/2000/svg"> <path d="M165.9 397.4c0 2-2.3 3.6-5.2 3.6-3.3.3-5.6-1.3-5.6-3.6 0-2 2.3-3.6 5.2-3.6 3-.3 5.6 1.3 5.6 3.6zm-31.1-4.5c-.7 2 1.3 4.3 4.3 4.9 2.6 1 5.6 0 6.2-2s-1.3-4.3-4.3-5.2c-2.6-.7-5.5.3-6.2 2.3zm44.2-1.7c-2.9.7-4.9 2.6-4.6 4.9.3 2 2.9 3.3 5.9 2.6 2.9-.7 4.9-2.6 4.6-4.6-.3-1.9-3-3.2-5.9-2.9zM244.8 8C106.1 8 0 113.3 0 252c0 110.9 69.8 205.8 169.5 239.2 12.8 2.3 17.3-5.6 17.3-12.1 0-6.2-.3-40.4-.3-61.4 0 0-70 15-84.7-29.8 0 0-11.4-29.1-27.8-36.6 0 0-22.9-15.7 1.6-15.4 0 0 24.9 2 38.6 25.8 21.9 38.6 58.6 27.5 72.9 20.9 2.3-16 8.8-27.1 16-33.7-55.9-6.2-112.3-14.3-112.3-110.5 0-27.5 7.6-41.3 23.6-58.9-2.6-6.5-11.1-33.3 2.6-67.9 20.9-6.5 69 27 69 27 20-5.6 41.5-8.5 62.8-8.5s42.8 2.9 62.8 8.5c0 0 48.1-33.6 69-27 13.7 34.7 5.2 61.4 2.6 67.9 16 17.7 25.8 31.5 25.8 58.9 0 96.5-58.9 104.2-114.8 110.5 9.2 7.9 17 22.9 17 46.4 0 33.7-.3 75.4-.3 83.6 0 6.5 4.6 14.4 17.3 12.1C428.2 457.8 496 362.9 496 252 496 113.3 383.5 8 244.8 8zM97.2 352.9c-1.3 1-1 3.3.7 5.2 1.6 1.6 3.9 2.3 5.2 1 1.3-1 1-3.3-.7-5.2-1.6-1.6-3.9-2.3-5.2-1zm-10.8-8.1c-.7 1.3.3 2.9 2.3 3.9 1.6 1 3.6.7 4.3-.7.7-1.3-.3-2.9-2.3-3.9-2-.6-3.6-.3-4.3.7zm32.4 35.6c-1.6 1.3-1 4.3 1.3 6.2 2.3 2.3 5.2 2.6 6.5 1 1.3-1.3.7-4.3-1.3-6.2-2.2-2.3-5.2-2.6-6.5-1zm-11.4-14.7c-1.6 1-1.6 3.6 0 5.9 1.6 2.3 4.3 3.3 5.6 2.3 1.6-1.3 1.6-3.9 0-6.2-1.4-2.3-4-3.3-5.6-2z"></path></svg>](https://github.com/spiousas) [<svg viewBox="0 0 512 512" style="height:1em;position:relative;display:inline-block;top:.1em;fill:#A42339;" xmlns="http://www.w3.org/2000/svg"> <path d="M459.37 151.716c.325 4.548.325 9.097.325 13.645 0 138.72-105.583 298.558-298.558 298.558-59.452 0-114.68-17.219-161.137-47.106 8.447.974 16.568 1.299 25.34 1.299 49.055 0 94.213-16.568 130.274-44.832-46.132-.975-84.792-31.188-98.112-72.772 6.498.974 12.995 1.624 19.818 1.624 9.421 0 18.843-1.3 27.614-3.573-48.081-9.747-84.143-51.98-84.143-102.985v-1.299c13.969 7.797 30.214 12.67 47.431 13.319-28.264-18.843-46.781-51.005-46.781-87.391 0-19.492 5.197-37.36 14.294-52.954 51.655 63.675 129.3 105.258 216.365 109.807-1.624-7.797-2.599-15.918-2.599-24.04 0-57.828 46.782-104.934 104.934-104.934 30.213 0 57.502 12.67 76.67 33.137 23.715-4.548 46.456-13.32 66.599-25.34-7.798 24.366-24.366 44.833-46.132 57.827 21.117-2.273 41.584-8.122 60.426-16.243-14.292 20.791-32.161 39.308-52.628 54.253z"></path></svg>](https://twitter.com/Spiousas) Pablo Etchemendy [<svg viewBox="0 0 496 512" style="height:1em;position:relative;display:inline-block;top:.1em;fill:black;" xmlns="http://www.w3.org/2000/svg"> <path d="M165.9 397.4c0 2-2.3 3.6-5.2 3.6-3.3.3-5.6-1.3-5.6-3.6 0-2 2.3-3.6 5.2-3.6 3-.3 5.6 1.3 5.6 3.6zm-31.1-4.5c-.7 2 1.3 4.3 4.3 4.9 2.6 1 5.6 0 6.2-2s-1.3-4.3-4.3-5.2c-2.6-.7-5.5.3-6.2 2.3zm44.2-1.7c-2.9.7-4.9 2.6-4.6 4.9.3 2 2.9 3.3 5.9 2.6 2.9-.7 4.9-2.6 4.6-4.6-.3-1.9-3-3.2-5.9-2.9zM244.8 8C106.1 8 0 113.3 0 252c0 110.9 69.8 205.8 169.5 239.2 12.8 2.3 17.3-5.6 17.3-12.1 0-6.2-.3-40.4-.3-61.4 0 0-70 15-84.7-29.8 0 0-11.4-29.1-27.8-36.6 0 0-22.9-15.7 1.6-15.4 0 0 24.9 2 38.6 25.8 21.9 38.6 58.6 27.5 72.9 20.9 2.3-16 8.8-27.1 16-33.7-55.9-6.2-112.3-14.3-112.3-110.5 0-27.5 7.6-41.3 23.6-58.9-2.6-6.5-11.1-33.3 2.6-67.9 20.9-6.5 69 27 69 27 20-5.6 41.5-8.5 62.8-8.5s42.8 2.9 62.8 8.5c0 0 48.1-33.6 69-27 13.7 34.7 5.2 61.4 2.6 67.9 16 17.7 25.8 31.5 25.8 58.9 0 96.5-58.9 104.2-114.8 110.5 9.2 7.9 17 22.9 17 46.4 0 33.7-.3 75.4-.3 83.6 0 6.5 4.6 14.4 17.3 12.1C428.2 457.8 496 362.9 496 252 496 113.3 383.5 8 244.8 8zM97.2 352.9c-1.3 1-1 3.3.7 5.2 1.6 1.6 3.9 2.3 5.2 1 1.3-1 1-3.3-.7-5.2-1.6-1.6-3.9-2.3-5.2-1zm-10.8-8.1c-.7 1.3.3 2.9 2.3 3.9 1.6 1 3.6.7 4.3-.7.7-1.3-.3-2.9-2.3-3.9-2-.6-3.6-.3-4.3.7zm32.4 35.6c-1.6 1.3-1 4.3 1.3 6.2 2.3 2.3 5.2 2.6 6.5 1 1.3-1.3.7-4.3-1.3-6.2-2.2-2.3-5.2-2.6-6.5-1zm-11.4-14.7c-1.6 1-1.6 3.6 0 5.9 1.6 2.3 4.3 3.3 5.6 2.3 1.6-1.3 1.6-3.9 0-6.2-1.4-2.3-4-3.3-5.6-2z"></path></svg>](https://github.com/https://github.com/petcheme) [<svg viewBox="0 0 512 512" style="height:1em;position:relative;display:inline-block;top:.1em;fill:#black;" xmlns="http://www.w3.org/2000/svg"> <path d="M459.37 151.716c.325 4.548.325 9.097.325 13.645 0 138.72-105.583 298.558-298.558 298.558-59.452 0-114.68-17.219-161.137-47.106 8.447.974 16.568 1.299 25.34 1.299 49.055 0 94.213-16.568 130.274-44.832-46.132-.975-84.792-31.188-98.112-72.772 6.498.974 12.995 1.624 19.818 1.624 9.421 0 18.843-1.3 27.614-3.573-48.081-9.747-84.143-51.98-84.143-102.985v-1.299c13.969 7.797 30.214 12.67 47.431 13.319-28.264-18.843-46.781-51.005-46.781-87.391 0-19.492 5.197-37.36 14.294-52.954 51.655 63.675 129.3 105.258 216.365 109.807-1.624-7.797-2.599-15.918-2.599-24.04 0-57.828 46.782-104.934 104.934-104.934 30.213 0 57.502 12.67 76.67 33.137 23.715-4.548 46.456-13.32 66.599-25.34-7.798 24.366-24.366 44.833-46.132 57.827 21.117-2.273 41.584-8.122 60.426-16.243-14.292 20.791-32.161 39.308-52.628 54.253z"></path></svg>](https://twitter.com/petcheme) **Agosto 2021** --- class: left, top, highlight-last-item # ggplot2 📈 <img src="https://raw.githubusercontent.com/rstudio/hex-stickers/master/PNG/ggplot2.png" width="20%" style="display: block; margin: auto;" /> En esta breve presentación vamos a hablar un poco de geometrías más complejas, como algunas **geoms_** y **stats_** --- class: left, top, highlight-last-item # geom_bar Con **geom_col()** hay que calcular la media antes. .pull-left[ ```r penguins %>% group_by(species) %>% summarise(mMass = mean(body_mass_g, na.rm = TRUE)) %>% ggplot(aes(x = species, y = mMass, fill = species)) + * geom_col() ``` ] .pull-right[ <img src="ggplot_2_files/figure-html/bar1-out-1.png" width="60%" style="display: block; margin: auto;" /> ] Usando **geom_bar()** se calcula la media de la magnitud en **y** para cada valor de **x** .pull-left[ ```r penguins %>% ggplot(aes(x = species, y = body_mass_g, fill = species)) + * geom_bar(fun = "mean", * stat = "summary") ``` ] .pull-right[ <img src="ggplot_2_files/figure-html/bar2-out-1.png" width="60%" style="display: block; margin: auto;" /> ] --- class: left, top, highlight-last-item # stat_count **stat_count** cumple la misma tarea que **geom_bar()** pero contando los casos .pull-left[ ```r penguins %>% group_by(species) %>% summarise(N = n()) %>% ggplot(aes(x = species, y = N, fill = species)) + * geom_col() ``` ] .pull-right[ <img src="ggplot_2_files/figure-html/count1-out-1.png" width="60%" style="display: block; margin: auto;" /> ] Podemos reemplazar ese pipe con esto: .pull-left[ ```r penguins %>% ggplot(aes(x = species, fill = species)) + * stat_count() ``` ] .pull-right[ <img src="ggplot_2_files/figure-html/count-out-1.png" width="60%" style="display: block; margin: auto;" /> ] --- class: left, top, highlight-last-item # stat_summary Usando **stat_summary()** agrega de alguna forma la magnitud en **y** para cada valor de **x** Por ejemplo, podemos calcular la media y el error estándar: .pull-left[ ```r penguins %>% ggplot(aes(x = species, y = body_mass_g, color = species)) + * stat_summary(fun.data = mean_se) ``` ] .pull-right[ <img src="ggplot_2_files/figure-html/summary1-out-1.png" width="55%" style="display: block; margin: auto;" /> ] O en formato barra: .pull-left[ ```r penguins %>% ggplot(aes(x = species, y = body_mass_g, fill = species)) + * stat_summary(fun.data = mean_se, * geom = "bar") + * stat_summary(fun.data = mean_se, * geom = "errorbar") ``` ] .pull-right[ <img src="ggplot_2_files/figure-html/summary2-out-1.png" width="55%" style="display: block; margin: auto;" /> ] --- class: left, top, highlight-last-item # geom_boxplot Un boxplot muestra de forma compacta la distribución de una variable continua. Es posible visualizar la **mediana**, el **IQR** y los **Outliers** .pull-left[ ```r penguins %>% ggplot(aes(x = species, y = body_mass_g, color = species)) + * geom_boxplot() ``` ] .pull-right[ <img src="ggplot_2_files/figure-html/boxplot1-out-1.png" width="55%" style="display: block; margin: auto;" /> ] También lo podemos usar para ver los datos y las estadísticas descriptivas .pull-left[ ```r penguins %>% ggplot(aes(x = species, y = body_mass_g, color = species)) + * geom_boxplot(outlier.shape = NA) + * geom_jitter(width = 0.2) ``` ] .pull-right[ <img src="ggplot_2_files/figure-html/boxplot2-out-1.png" width="55%" style="display: block; margin: auto;" /> ] ??? Mencionar que se puede ajustar el criterio de outliers en base al IQR --- class: left, top, highlight-last-item # geom_violin **geom_violin()** nos permite graficar un **violin plot** de los datos Un **Violin plot** es una forma compacta de mostrar una distribución continua Es una mezcla entre un **geom_boxplot()** y **geom_density()** .pull-left[ ```r penguins %>% ggplot(aes(x = species, y = body_mass_g, fill = species)) + * geom_violin() ``` ] .pull-right[ <img src="ggplot_2_files/figure-html/violin-out-1.png" width="80%" style="display: block; margin: auto;" /> ] Combinado con los datos crudos podemos hacer un **raincloud plot**, pero esa es historia para más adelnte --- class: left, top, highlight-last-item # geom_smooth **geom_smooth** ayuda a ver patrones en nubes de puntos suporponiendo una capa suavizada .pull-left[ ```r penguins %>% filter(species == "Adelie") %>% ggplot(aes(x = bill_length_mm, y = bill_depth_mm)) + geom_point() + * geom_smooth() ``` ] .pull-right[ <img src="ggplot_2_files/figure-html/smooth1-out-1.png" width="55%" style="display: block; margin: auto;" /> ] Se puede forzar la **geometrìa** de esa capa .pull-left[ ```r penguins %>% filter(species == "Adelie") %>% ggplot(aes(x = bill_length_mm, y = bill_depth_mm)) + geom_point() + * geom_smooth(se = FALSE, * method = lm) ``` ] .pull-right[ <img src="ggplot_2_files/figure-html/smooth2-out-1.png" width="55%" style="display: block; margin: auto;" /> ] --- class: left, top, highlight-last-item # geom_hex **geom_hex** divide el plano en polígonos regulares y cuenta la candidad de casos en cada **bin** Es una especie de **histograma bidimensional** o **heathmap discreto** ```r penguins %>% filter(species == "Adelie") %>% ggplot(aes(x = bill_length_mm, y = bill_depth_mm)) + * geom_hex(binwidth = c(1, 0.5)) ``` <img src="ggplot_2_files/figure-html/hex-out-1.png" width="40%" style="display: block; margin: auto;" /> --- class: left, top, highlight-last-item # Para seguir investigando... Pueden seguir investigando más **geoms**, capas y escalas [acá](https://ggplot2.tidyverse.org/reference/) 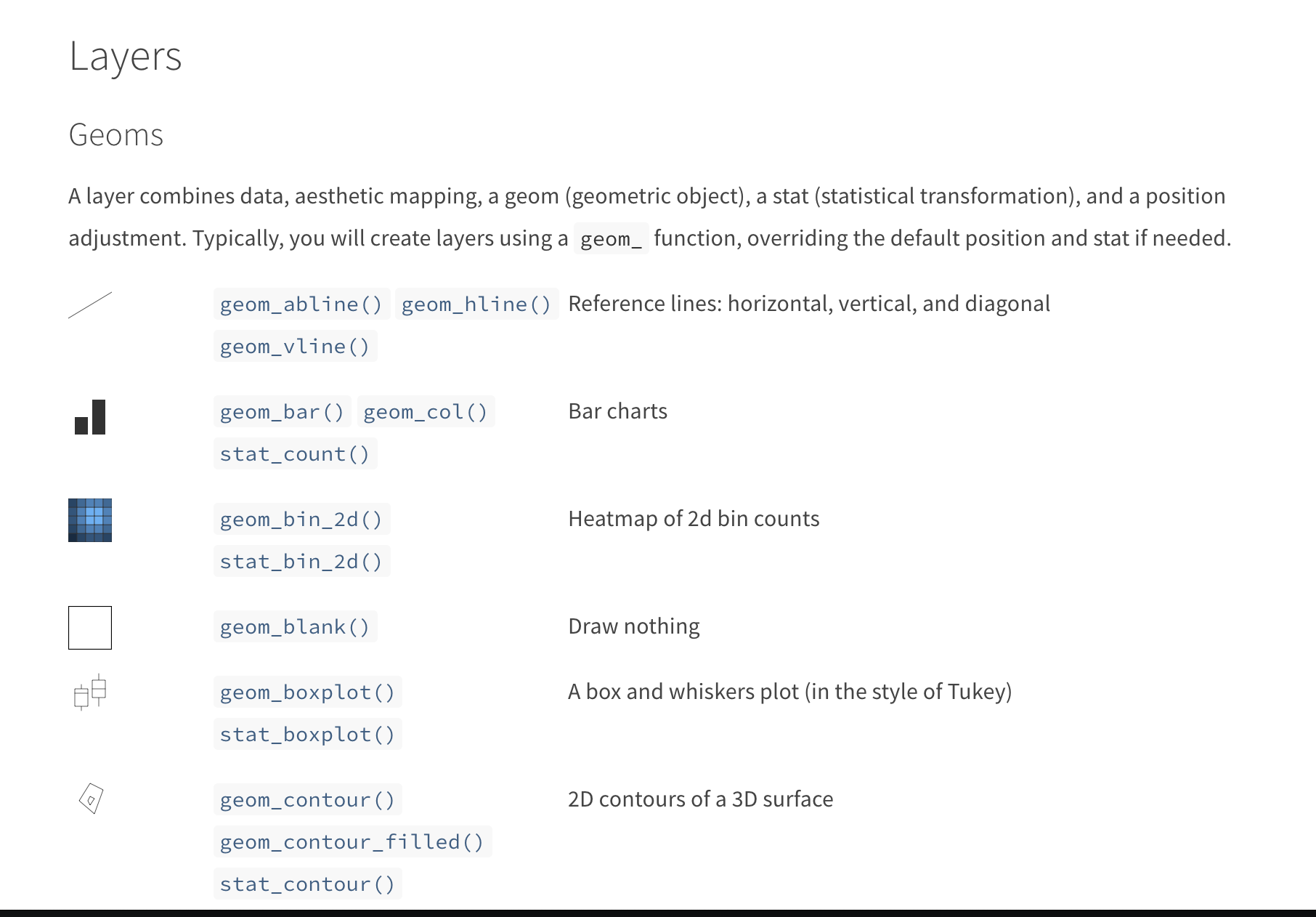 --- class: center, top # Referencias .left[.big[ - Nordmann, E., McAleer, P., Toivo, W., Paterson, H., & DeBruine, L. (2021). Data visualisation using R, for researchers who don't use R. - Wickham, H. (2011). ggplot2. Wiley Interdisciplinary Reviews: Computational Statistics, 3(2), 180-185. ]]