class: center, middle # Inferencia con regresión múltiple ### Análisis estadístico utilizando R <img src="https://miro.medium.com/max/1000/1*ZhYNqU2y96_f3QkWq9oiWQ.jpeg" width="30%" /> UNQ UNTreF CONICET Ignacio Spiousas [<svg viewBox="0 0 496 512" style="height:1em;position:relative;display:inline-block;top:.1em;fill:#A42339;" xmlns="http://www.w3.org/2000/svg"> <path d="M165.9 397.4c0 2-2.3 3.6-5.2 3.6-3.3.3-5.6-1.3-5.6-3.6 0-2 2.3-3.6 5.2-3.6 3-.3 5.6 1.3 5.6 3.6zm-31.1-4.5c-.7 2 1.3 4.3 4.3 4.9 2.6 1 5.6 0 6.2-2s-1.3-4.3-4.3-5.2c-2.6-.7-5.5.3-6.2 2.3zm44.2-1.7c-2.9.7-4.9 2.6-4.6 4.9.3 2 2.9 3.3 5.9 2.6 2.9-.7 4.9-2.6 4.6-4.6-.3-1.9-3-3.2-5.9-2.9zM244.8 8C106.1 8 0 113.3 0 252c0 110.9 69.8 205.8 169.5 239.2 12.8 2.3 17.3-5.6 17.3-12.1 0-6.2-.3-40.4-.3-61.4 0 0-70 15-84.7-29.8 0 0-11.4-29.1-27.8-36.6 0 0-22.9-15.7 1.6-15.4 0 0 24.9 2 38.6 25.8 21.9 38.6 58.6 27.5 72.9 20.9 2.3-16 8.8-27.1 16-33.7-55.9-6.2-112.3-14.3-112.3-110.5 0-27.5 7.6-41.3 23.6-58.9-2.6-6.5-11.1-33.3 2.6-67.9 20.9-6.5 69 27 69 27 20-5.6 41.5-8.5 62.8-8.5s42.8 2.9 62.8 8.5c0 0 48.1-33.6 69-27 13.7 34.7 5.2 61.4 2.6 67.9 16 17.7 25.8 31.5 25.8 58.9 0 96.5-58.9 104.2-114.8 110.5 9.2 7.9 17 22.9 17 46.4 0 33.7-.3 75.4-.3 83.6 0 6.5 4.6 14.4 17.3 12.1C428.2 457.8 496 362.9 496 252 496 113.3 383.5 8 244.8 8zM97.2 352.9c-1.3 1-1 3.3.7 5.2 1.6 1.6 3.9 2.3 5.2 1 1.3-1 1-3.3-.7-5.2-1.6-1.6-3.9-2.3-5.2-1zm-10.8-8.1c-.7 1.3.3 2.9 2.3 3.9 1.6 1 3.6.7 4.3-.7.7-1.3-.3-2.9-2.3-3.9-2-.6-3.6-.3-4.3.7zm32.4 35.6c-1.6 1.3-1 4.3 1.3 6.2 2.3 2.3 5.2 2.6 6.5 1 1.3-1.3.7-4.3-1.3-6.2-2.2-2.3-5.2-2.6-6.5-1zm-11.4-14.7c-1.6 1-1.6 3.6 0 5.9 1.6 2.3 4.3 3.3 5.6 2.3 1.6-1.3 1.6-3.9 0-6.2-1.4-2.3-4-3.3-5.6-2z"></path></svg>](https://github.com/spiousas) [<svg viewBox="0 0 512 512" style="height:1em;position:relative;display:inline-block;top:.1em;fill:#A42339;" xmlns="http://www.w3.org/2000/svg"> <path d="M459.37 151.716c.325 4.548.325 9.097.325 13.645 0 138.72-105.583 298.558-298.558 298.558-59.452 0-114.68-17.219-161.137-47.106 8.447.974 16.568 1.299 25.34 1.299 49.055 0 94.213-16.568 130.274-44.832-46.132-.975-84.792-31.188-98.112-72.772 6.498.974 12.995 1.624 19.818 1.624 9.421 0 18.843-1.3 27.614-3.573-48.081-9.747-84.143-51.98-84.143-102.985v-1.299c13.969 7.797 30.214 12.67 47.431 13.319-28.264-18.843-46.781-51.005-46.781-87.391 0-19.492 5.197-37.36 14.294-52.954 51.655 63.675 129.3 105.258 216.365 109.807-1.624-7.797-2.599-15.918-2.599-24.04 0-57.828 46.782-104.934 104.934-104.934 30.213 0 57.502 12.67 76.67 33.137 23.715-4.548 46.456-13.32 66.599-25.34-7.798 24.366-24.366 44.833-46.132 57.827 21.117-2.273 41.584-8.122 60.426-16.243-14.292 20.791-32.161 39.308-52.628 54.253z"></path></svg>](https://twitter.com/Spiousas) Pablo Etchemendy [<svg viewBox="0 0 496 512" style="height:1em;position:relative;display:inline-block;top:.1em;fill:black;" xmlns="http://www.w3.org/2000/svg"> <path d="M165.9 397.4c0 2-2.3 3.6-5.2 3.6-3.3.3-5.6-1.3-5.6-3.6 0-2 2.3-3.6 5.2-3.6 3-.3 5.6 1.3 5.6 3.6zm-31.1-4.5c-.7 2 1.3 4.3 4.3 4.9 2.6 1 5.6 0 6.2-2s-1.3-4.3-4.3-5.2c-2.6-.7-5.5.3-6.2 2.3zm44.2-1.7c-2.9.7-4.9 2.6-4.6 4.9.3 2 2.9 3.3 5.9 2.6 2.9-.7 4.9-2.6 4.6-4.6-.3-1.9-3-3.2-5.9-2.9zM244.8 8C106.1 8 0 113.3 0 252c0 110.9 69.8 205.8 169.5 239.2 12.8 2.3 17.3-5.6 17.3-12.1 0-6.2-.3-40.4-.3-61.4 0 0-70 15-84.7-29.8 0 0-11.4-29.1-27.8-36.6 0 0-22.9-15.7 1.6-15.4 0 0 24.9 2 38.6 25.8 21.9 38.6 58.6 27.5 72.9 20.9 2.3-16 8.8-27.1 16-33.7-55.9-6.2-112.3-14.3-112.3-110.5 0-27.5 7.6-41.3 23.6-58.9-2.6-6.5-11.1-33.3 2.6-67.9 20.9-6.5 69 27 69 27 20-5.6 41.5-8.5 62.8-8.5s42.8 2.9 62.8 8.5c0 0 48.1-33.6 69-27 13.7 34.7 5.2 61.4 2.6 67.9 16 17.7 25.8 31.5 25.8 58.9 0 96.5-58.9 104.2-114.8 110.5 9.2 7.9 17 22.9 17 46.4 0 33.7-.3 75.4-.3 83.6 0 6.5 4.6 14.4 17.3 12.1C428.2 457.8 496 362.9 496 252 496 113.3 383.5 8 244.8 8zM97.2 352.9c-1.3 1-1 3.3.7 5.2 1.6 1.6 3.9 2.3 5.2 1 1.3-1 1-3.3-.7-5.2-1.6-1.6-3.9-2.3-5.2-1zm-10.8-8.1c-.7 1.3.3 2.9 2.3 3.9 1.6 1 3.6.7 4.3-.7.7-1.3-.3-2.9-2.3-3.9-2-.6-3.6-.3-4.3.7zm32.4 35.6c-1.6 1.3-1 4.3 1.3 6.2 2.3 2.3 5.2 2.6 6.5 1 1.3-1.3.7-4.3-1.3-6.2-2.2-2.3-5.2-2.6-6.5-1zm-11.4-14.7c-1.6 1-1.6 3.6 0 5.9 1.6 2.3 4.3 3.3 5.6 2.3 1.6-1.3 1.6-3.9 0-6.2-1.4-2.3-4-3.3-5.6-2z"></path></svg>](https://github.com/https://github.com/petcheme) [<svg viewBox="0 0 512 512" style="height:1em;position:relative;display:inline-block;top:.1em;fill:#black;" xmlns="http://www.w3.org/2000/svg"> <path d="M459.37 151.716c.325 4.548.325 9.097.325 13.645 0 138.72-105.583 298.558-298.558 298.558-59.452 0-114.68-17.219-161.137-47.106 8.447.974 16.568 1.299 25.34 1.299 49.055 0 94.213-16.568 130.274-44.832-46.132-.975-84.792-31.188-98.112-72.772 6.498.974 12.995 1.624 19.818 1.624 9.421 0 18.843-1.3 27.614-3.573-48.081-9.747-84.143-51.98-84.143-102.985v-1.299c13.969 7.797 30.214 12.67 47.431 13.319-28.264-18.843-46.781-51.005-46.781-87.391 0-19.492 5.197-37.36 14.294-52.954 51.655 63.675 129.3 105.258 216.365 109.807-1.624-7.797-2.599-15.918-2.599-24.04 0-57.828 46.782-104.934 104.934-104.934 30.213 0 57.502 12.67 76.67 33.137 23.715-4.548 46.456-13.32 66.599-25.34-7.798 24.366-24.366 44.833-46.132 57.827 21.117-2.273 41.584-8.122 60.426-16.243-14.292 20.791-32.161 39.308-52.628 54.253z"></path></svg>](https://twitter.com/petcheme) 2021-08-20 --- class: left, top, highlight-last-item # Ahora sí .center[ 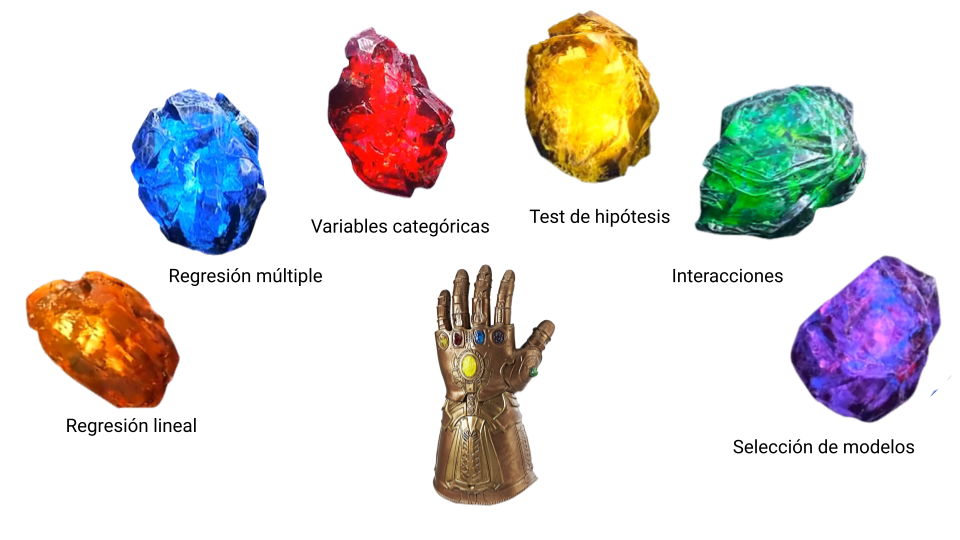 ] --- class: left, top, highlight-last-item # Los pingüinos de nuevo Volvamos al modelo con **bill_depth_mm** y **species** (Adelie y Gentoo) `$$\hat{Peso} = b_0 + b_1 AnchoPico + b_2 EspecieGentoo$$` .pull-left[ ```r penguins_adelie_gentoo <- penguins %>% drop_na() %>% filter(species %in% c("Adelie", "Gentoo")) m2 <- penguins_adelie_gentoo%>% lm(body_mass_g ~ bill_depth_mm + species, .) summary(m2) ``` ``` ## ## Call: ## lm(formula = body_mass_g ~ bill_depth_mm + species, data = .) ## ## Residuals: ## Min 1Q Median 3Q Max ## -849.44 -259.45 -31.32 255.49 1195.69 ## ## Coefficients: ## Estimate Std. Error t value Pr(>|t|) ## (Intercept) -1249.97 375.83 -3.326 0.00101 ** ## bill_depth_mm 270.13 20.42 13.231 < 2e-16 *** ## speciesGentoo 2291.37 82.34 27.829 < 2e-16 *** ## --- ## Signif. codes: 0 '***' 0.001 '**' 0.01 '*' 0.05 '.' 0.1 ' ' 1 ## ## Residual standard error: 371 on 262 degrees of freedom ## Multiple R-squared: 0.8062, Adjusted R-squared: 0.8048 ## F-statistic: 545.1 on 2 and 262 DF, p-value: < 2.2e-16 ``` ] .pull-right[ A diferencia de cuando teníamos un predictor donde: `\(H_0:\beta_1=0\)` Los p-values que reporta `summary()` son contra la hipótesis nula: `\(H_0:\beta_i=0\)` dadas todas las otras variables del modelos. Por ejemplo: El efecto del parámetro `speciesGentoo` tiene un `p<2e-16` `\(H_0:\beta_2=0\)` dado que término de `bill_depth_mm` es incluido en el modelo. y el efecto del parámetro `bill_depth_mm` tiene un `p<2e-16` `\(H_0:\beta_1=0\)` dado que término de `speciesGentoo` es incluido en el modelo. ] --- class: left, top, highlight-last-item # Los pingüinos de nuevo Ageguemos todas las especies `$$\hat{Peso} = b_0 + b_1 AnchoPico + b_2 EspecieChinstrap + b_3 EspecieGentoo$$` .pull-left[ ```r m2_full <- penguins %>% drop_na() %>% lm(body_mass_g ~ bill_depth_mm + species, .) summary(m2_full) ``` ``` ## ## Call: ## lm(formula = body_mass_g ~ bill_depth_mm + species, data = .) ## ## Residuals: ## Min 1Q Median 3Q Max ## -846.01 -261.75 -30.43 232.36 1185.55 ## ## Coefficients: ## Estimate Std. Error t value Pr(>|t|) ## (Intercept) -1000.846 324.968 -3.080 0.00225 ** ## bill_depth_mm 256.551 17.637 14.546 < 2e-16 *** ## speciesChinstrap 8.111 52.874 0.153 0.87817 ## speciesGentoo 2245.878 73.956 30.368 < 2e-16 *** ## --- ## Signif. codes: 0 '***' 0.001 '**' 0.01 '*' 0.05 '.' 0.1 ' ' 1 ## ## Residual standard error: 360 on 329 degrees of freedom ## Multiple R-squared: 0.8019, Adjusted R-squared: 0.8001 ## F-statistic: 443.9 on 3 and 329 DF, p-value: < 2.2e-16 ``` ] .pull-right[ Vamos que `speciesChinstrap` no es significativo. <img src="linear_models_4_files/figure-html/unnamed-chunk-3-1.png" width="80%" style="display: block; margin: auto;" /> ] --- class: left, top, highlight-last-item # Estadístico F .pull-left[ .big[¿Qué pasa si lo que queremos ver es el efecto combinado de especie?] O sea, el efecto combinado de `\(\beta_2\)` y `\(\beta_2\)` dado `\(\beta_1\)` Para esto vamos a usar la estadística **F** `$$F = \frac{(SSR_{restringida}-SSR_{sinrestringir})/q}{SSR_{sinrestringir}/(n-k-1)}$$` `\(SSR_{restringida}\)` ```r SSR_res <- sum(resid(m2_full)^2) SSR_res ``` ``` ## [1] 42644614 ``` `\(SSR_{sinrestringir}\)` ```r m2 <- penguins %>% drop_na() %>% lm(body_mass_g ~ bill_depth_mm, .) SSR_unres <- sum(resid(m2)^2) SSR_unres ``` ``` ## [1] 167300074 ``` ] .pull-right[ ```r q <- 2 n <- 333 k <- 1 F <- ((SSR_res-SSR_unres)/q)/(SSR_res/(n-k-1)) F ``` ``` ## [1] -483.7769 ``` Tranquilos que se puede calcular usando **R** ```r #library(car) anova(m2_full, m2) ``` ``` ## Analysis of Variance Table ## ## Model 1: body_mass_g ~ bill_depth_mm + species ## Model 2: body_mass_g ~ bill_depth_mm ## Res.Df RSS Df Sum of Sq F Pr(>F) ## 1 329 42644614 ## 2 331 167300074 -2 -124655460 480.85 < 2.2e-16 *** ## --- ## Signif. codes: 0 '***' 0.001 '**' 0.01 '*' 0.05 '.' 0.1 ' ' 1 ``` ] --- class: left, top, highlight-last-item # Los efectos Si queremos ver todos los efectos combinados ```r Anova(m2_full, type = 3) ``` ``` ## Anova Table (Type III tests) ## ## Response: body_mass_g ## Sum Sq Df F value Pr(>F) ## (Intercept) 1229481 1 9.4853 0.002246 ** ## bill_depth_mm 27424833 1 211.5805 < 2.2e-16 *** ## species 124655460 2 480.8538 < 2.2e-16 *** ## Residuals 42644614 329 ## --- ## Signif. codes: 0 '***' 0.001 '**' 0.01 '*' 0.05 '.' 0.1 ' ' 1 ``` En este caso, cada efecto se compara con el modelo sin ese predictor Por ejemplo. la `\(H_0\)` de `bill_depth_mm` es un modelo con `intercept` y `species` --- class: left, top, highlight-last-item # Interacciones `$$\hat{Peso} = b_0 + b_1 AnchoPico + b_2 AnchoAleta + b_3 AnchoPico \times AnchoAleta$$` ```r m3 <- penguins %>% drop_na() %>% lm(body_mass_g ~ bill_depth_mm * flipper_length_mm, .) Anova(m3, type = 3) ``` ``` ## Anova Table (Type III tests) ## ## Response: body_mass_g ## Sum Sq Df F value Pr(>F) ## (Intercept) 8724147 1 63.995 2.152e-14 *** ## bill_depth_mm 6140161 1 45.040 8.457e-11 *** ## flipper_length_mm 10771476 1 79.013 < 2.2e-16 *** ## bill_depth_mm:flipper_length_mm 6021789 1 44.172 1.250e-10 *** ## Residuals 44851286 329 ## --- ## Signif. codes: 0 '***' 0.001 '**' 0.01 '*' 0.05 '.' 0.1 ' ' 1 ``` --- class: left, top, highlight-last-item # Dos variables categóricas con interacción `$$\hat{Peso} = b_0 + b_1 Sexo + b_2 Especie + b_3 Sexo \times Especie$$` <img src="linear_models_4_files/figure-html/unnamed-chunk-10-1.png" width="40%" style="display: block; margin: auto;" /> --- class: left, top, highlight-last-item # Dos variables categóricas con interacción `$$\hat{Peso} = b_0 + b_1 Sexo + b_2 Especie + b_3 Sexo \times Especie$$` ```r m4 <- penguins %>% drop_na() %>% lm(body_mass_g ~ sex * species, .) m4 ``` ``` ## ## Call: ## lm(formula = body_mass_g ~ sex * species, data = .) ## ## Coefficients: ## (Intercept) sexmale speciesChinstrap ## 3368.8 674.7 158.4 ## speciesGentoo sexmale:speciesChinstrap sexmale:speciesGentoo ## 1310.9 -262.9 130.4 ``` `$$\hat{Peso} = 3368.8 + 674.7·SexoM + 158.4·EspecieChin + 1310.9·EspecieGentoo ...$$` `$$...-262.9·SexoM \times EspecieChinstrap + 130.4·SexoM \times EspecieGentoo$$` .pull-left[ Un pingüino **hembra** y **Adelie** ```r peso <- 3368.8 + 674.7 * 0 + 158.4 * 0 + 1310.9 * 0 - 262.9 * 0 * 0 + 130.4 * 0 * 0 peso ``` ``` ## [1] 3368.8 ``` ] .pull-right[ Un pingüino **macho** y **Gentoo** ```r peso <- 3368.8 + 674.7 * 1 + 158.4 * 0 + 1310.9 * 1 - 262.9 * 1 * 0 + 130.4 * 1 * 1 peso ``` ``` ## [1] 5484.8 ``` ] --- class: left, top, highlight-last-item # Dos variables categóricas con interacción Finalmente vamos a testear los **efectos conjuntos** de cada predictor: ```r Anova(m4, type = 3) ``` ``` ## Anova Table (Type III tests) ## ## Response: body_mass_g ## Sum Sq Df F value Pr(>F) ## (Intercept) 828480899 1 8654.649 < 2.2e-16 *** ## sex 16613442 1 173.551 < 2.2e-16 *** ## species 60350016 2 315.220 < 2.2e-16 *** ## sex:species 1676557 2 8.757 0.0001973 *** ## Residuals 31302628 327 ## --- ## Signif. codes: 0 '***' 0.001 '**' 0.01 '*' 0.05 '.' 0.1 ' ' 1 ``` -- .center[ 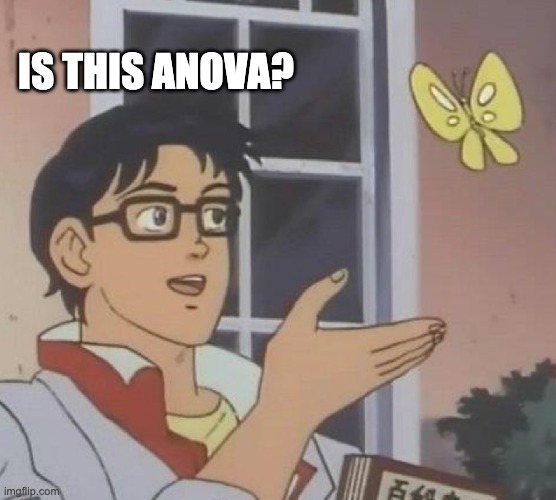 ] --- class: left, top, highlight-last-item .center[ 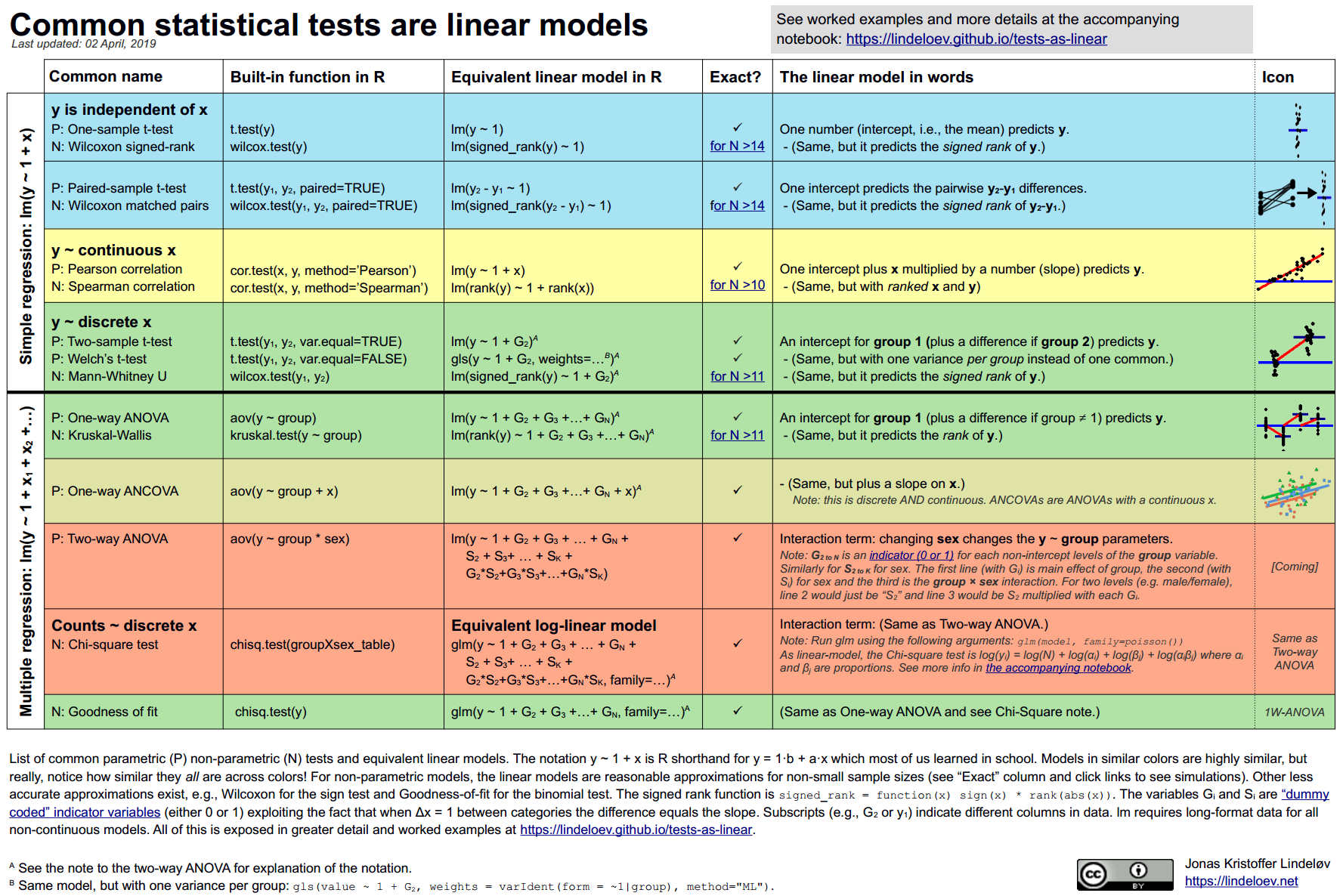 ] --- class: left, top, highlight-last-item # Resumen .huge[ Aprendimos a: - Evaluar el **efecto** de un predictor - Testear **hipótesis** sobre los predictores - Que la mayoría de los test estadísticos pueden pensarse como un modelo lineal ¿Qué pasa si las mediciones no son independientes? Por ejemplo, medidas repetidas, datos de un mismo grupo o camada, mediciones a lo largo del tiempo... No se preocupen, vienen los **Modelos Lineales de Efectos Mixtos** al rescate en las próximas clases ] --- class: center, top # Referencias .left[.big[ - Mine Çetinkaya-Rundel and Johanna Hardin (2021). Introduction to Modern Statistics. Openintro Project. https://openintro-ims.netlify.app/index.html. - Jonas Kristoffer Lindeløv. Common statistical tests are linear models (or: how to teach stats), https://lindeloev.github.io/tests-as-linear/ ]]